Whilst much computational work is undertaken to support, library design, virtual screening, hit selection and affinity optimisation the reality is that the most challenging issues to resolve in drug discovery often revolve around absorption, distribution, metabolism and excretion (ADME). Whilst we can measure the levels of parent drug in various medium tracking metabolic fate can often be a considerably more difficult proposition requiring significant resources. For this reason prediction of sites of metabolism has become the subject of current interest.
The challenge of the problem should not be under-estimated, there are multiple different potential enzymic reaction types, those that act directly on the drug (phase I) and those the further functionalize metabolites (phase II), any drug can be a substrate for multiple enzymes. Predictions involving QM calculations or docking to the flexible binding sites of cytochrome P450 enzymes require prohibitive computing resources.
FAME DOI is a collection of random forest models trained on a comprehensive and highly diverse data set of 20,000 small molecules annotated with their experimentally determined sites of metabolism taken from multiple species (rat, dog and human). In addition dedicated models are available to predict sites of metabolism of phase I and II processes. Remarkably this is achieved using only 7 easily calculated descriptors (Table 1), six interpretable atomic descriptors (encoding the element type, hybridization state, and electronic configuration of each atom) and one molecular descriptor (encoding the topological size of a molecule).
FAME 2 DOI builds on this work to improve accuracy, in addition FAME 2 uses a slightly modified version of the visualisation developed by Patrik Rydberg and implemented in SMARTCyp using ChemDoodle Web Components.
It is really useful to have two sites of metabolism tools available that use contrasting methodologies, FAME 2 using curated dataset of experimentally determined metabolism data to build a machine learning model using simple descriptors. In contrast SMARTCyp uses precomputed activation energies from density functional theory (DFT) calculations of model compounds. These are used to predict the reactivity of similar fragments within the target molecule the final score is modified to reflect the accessibility to the active site of the different CYP450 iso forms and improvements for N-oxidations of tertiary amines are included, specifically an empirical corrections to unlikely oxidations of tertiary alkylamines
In FAME 2 rather than using the simple random forest machine learning algorithm used in the original method, an extremely randomised trees approach is used DOI which is a computationally efficient classification algorithm. FAME used a set of 2D descriptors 7 easily calculated descriptors, six interpretable atomic descriptors (encoding the element type, hybridization state, and electronic configuration of each atom) and one molecular descriptor (encoding the topological size of a molecule). In contrast FAME 2 uses circular descriptions of atoms and their environments. As can be seen in the help message below it is possible to change the diameter of the atom encoding fingerprint from 1 to 6. The default ‘circCDKATF1′ is a model based on the atom itself and its immediate neighbors (atoms at most one bond away).
1 2 3 4 5 6 7 8 9 10 11 12 13 14 15 16 17 18 19 20 21 22 23 24 25 26 27 28 29 30 31 32 33 34 35 36 37 38 39 40 41 42 43 44 45 46 47 48 49 50 51 52 53 54 |
java -jar /Users/Username/Downloads/fame2/fame2.jar -h usage: fame2 [-h] [--version] [-m {circCDK_ATF_1,circCDK_4,circCDK_ATF_6}] [-s [SMILES [SMILES ...]]] [-o OUTPUT_DIRECTORY] [-p] [-c] [FILE [FILE ...]] This is fame2. It attempts to predict sites of metabolism for supplied chemical compounds. It includes extra trees models for regioselectivity prediction of some cytochrome P450 isoforms. positional arguments: FILE One or more SDF files with compounds to predict. One SDF can contain multiple compounds. All molecules should be neutral and have explicit hydrogens added prior to modelling. If there are still missing hydrogens, the software will try to add them automatically.Calculating spatial coordinates of atoms is not necessary. optional arguments: -h, --help show this help message and exit --version Show program version. -m {circCDK_ATF_1,circCDK_4,circCDK_ATF_6}, --model {circCDK_ATF_1,circCDK_4,circCDK_ATF_6} Model to use to generate predictions. Either the model with the best average performance ('circCDK_ATF_6') during the independent test set validation as performed in the original paper or one of the simpler models that were found to have comparable performance ('circCDK_ATF_1' and 'circCDK_4'). The 'circCDK_ATF_1' model is selected by default as it is expected to offer the best trade-off between generalization and accuracy. The number after the model code indicates how wide the encodedenvironment of an atom is. For example, the default 'circCDK_ATF_1' is a model based on the atom itself and its immediate neighbors (atoms at most one bond away). (default: circCDK_ATF_1) -s [SMILES [SMILES ...]], --smiles [SMILES [SMILES ...]] One or more SMILES strings of compounds to predict. All molecules should be neutral and have explicit hydrogens added prior to modelling. If there are still missing hydrogens, the software will try to add them automatically. -o OUTPUT_DIRECTORY, --output-directory OUTPUT_DIRECTORY The path to the output directory. If it doesn't exist, it will be created. (default: fame_results) -p, --depict-png Generates depictions of molecules with the predicted sites highlighted as PNG files in addition to the HTML output. (default: false) -c, --output-csv Saves calculated descriptors and predictions to CSV files. (default: false) |
The predictions are generated as a simple HTML page (shown below) which displays the structure of the compound with the predicted SoMs highlighted with yellow circles, moving the cursor over the structure reals the atom numbers that correspond to the numbers in the table.
FAME II Output
Produced: 2017-08-15_20-53-43.
Input file: [/Users/username/Desktop/fame2/example_compounds/tamoxifen.sdf].
Visualization:
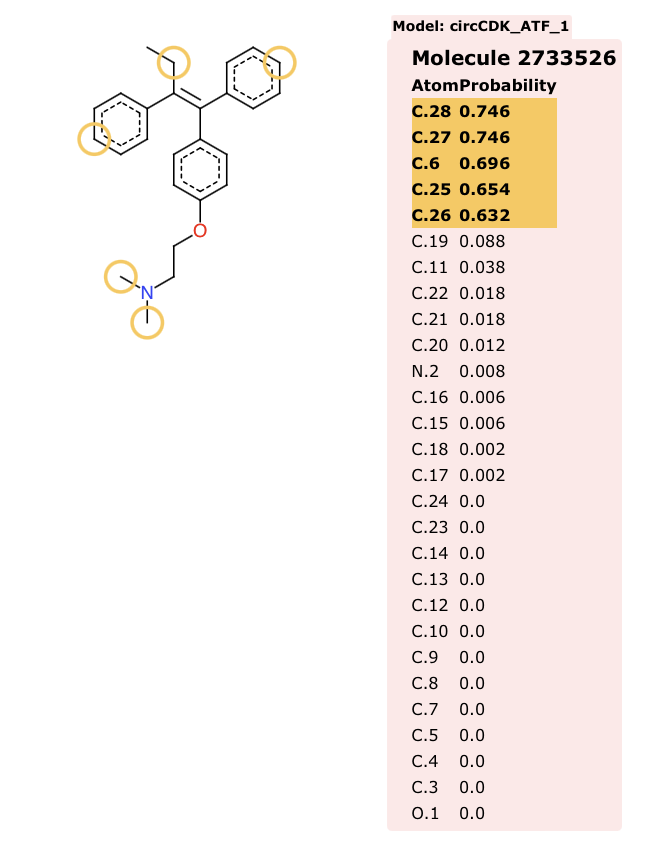
I also used SMARTCyp to predict the sites of metabolism for Tamoxifen, the results are very similar and predict the known routes of metabolism. In particular they flag the CYP2D6 mediated 4-hydroxylation to give the active metabolite 4-hydroxytamoxifen and the the demethylation sites.
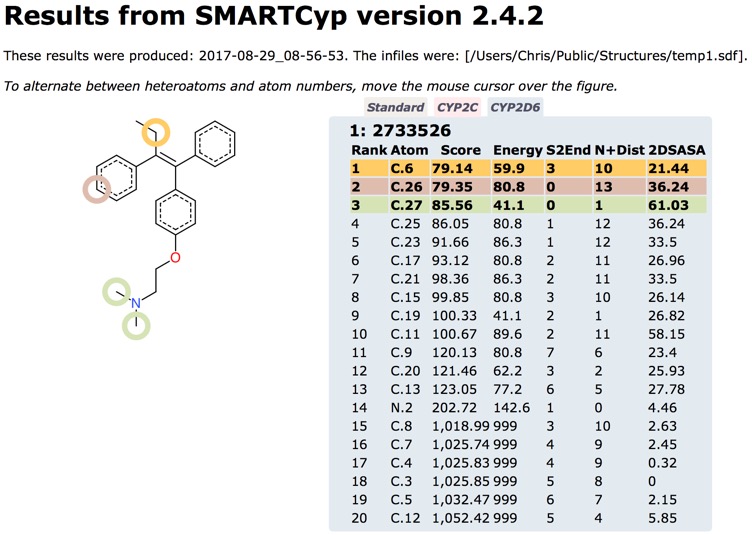
It is also possible to use SMILES as input
1 2 |
java -jar /Users/Chris/Desktop/fame2/fame2.jar -s 'CC/C(=C(\c1ccccc1)c1ccc(cc1)OCCN(C)C)c1ccccc1' |
I looked at the influence of the different models used to generate predictions.
1 2 3 4 5 6 7 8 9 10 11 12 13 14 15 16 17 18 19 20 21 22 23 24 25 26 27 28 29 30 31 32 33 34 35 36 37 38 39 40 |
MacPro:~ Chris$ java -jar /Users/Chris/Desktop/fame2/fame2.jar -s 'Cn1cc(cn1)c2ccc3nnc(n3n2)Sc4ccc5c(c4)cccn5' -m circCDK_ATF_1 Selected model: circCDK_ATF_1 Output Directory: fame_results Loading model... Processing: [Cn1cc(cn1)c2ccc3nnc(n3n2)Sc4ccc5c(c4)cccn5] Generating identifier for Cn1cc(cn1)c2ccc3nnc(n3n2)Sc4ccc5c(c4)cccn5: mol_1_1 ************** Processing molecule: mol_1_1 ************** WARNING: implicit hydrogens detected for molecule: mol_1_1 Making all hydrogens explicit... Explicit hydrogens in the original structure: 0 Added hydrogens: 13 Prediction and descriptor calculation finished (mol_1_1). Elapsed time: 1779.6517997 ms. ************** Done (mol_1_1) ************** MacPro:~ Chris$ java -jar /Users/Chris/Desktop/fame2/fame2.jar -s 'Cn1cc(cn1)c2ccc3nnc(n3n2)Sc4ccc5c(c4)cccn5' -m circCDK_4 Selected model: circCDK_4 Output Directory: fame_results Loading model... Processing: [Cn1cc(cn1)c2ccc3nnc(n3n2)Sc4ccc5c(c4)cccn5] Generating identifier for Cn1cc(cn1)c2ccc3nnc(n3n2)Sc4ccc5c(c4)cccn5: mol_1_1 ************** Processing molecule: mol_1_1 ************** WARNING: implicit hydrogens detected for molecule: mol_1_1 Making all hydrogens explicit... Explicit hydrogens in the original structure: 0 Added hydrogens: 13 Prediction and descriptor calculation finished (mol_1_1). Elapsed time: 2453.465267 ms. ************** Done (mol_1_1) ************** MacPro:~ Chris$ java -jar /Users/Chris/Desktop/fame2/fame2.jar -s 'Cn1cc(cn1)c2ccc3nnc(n3n2)Sc4ccc5c(c4)cccn5' -m circCDK_ATF_6 Selected model: circCDK_ATF_6 Output Directory: fame_results Loading model... Processing: [Cn1cc(cn1)c2ccc3nnc(n3n2)Sc4ccc5c(c4)cccn5] Generating identifier for Cn1cc(cn1)c2ccc3nnc(n3n2)Sc4ccc5c(c4)cccn5: mol_1_1 ************** Processing molecule: mol_1_1 ************** WARNING: implicit hydrogens detected for molecule: mol_1_1 Making all hydrogens explicit... Explicit hydrogens in the original structure: 0 Added hydrogens: 13 Prediction and descriptor calculation finished (mol_1_1). Elapsed time: 2632.7860776 ms. ************** Done (mol_1_1) ************** |
Whilst the default circCDKATF1 is the fastest I found instances where circCDKATF6 gave more accurate results as shown below.
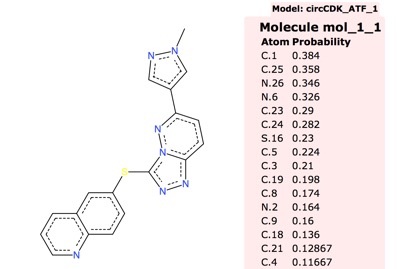
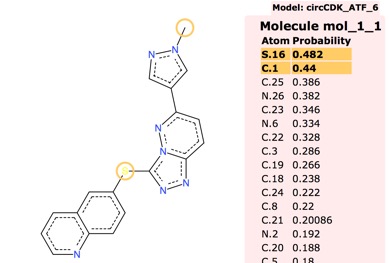
ummary
When I first reviewed FAME2 there were a couple of minor bugs, when I reported them to the developers the bugs were fixed and a new version of FAME2 was made available within a day, really impressive support! Unfortunately (unlike FAME1) FAME2 only predicts CYP450 mediated metabolism, apparently the non-CYP mediated metabolism data was not available to the author.